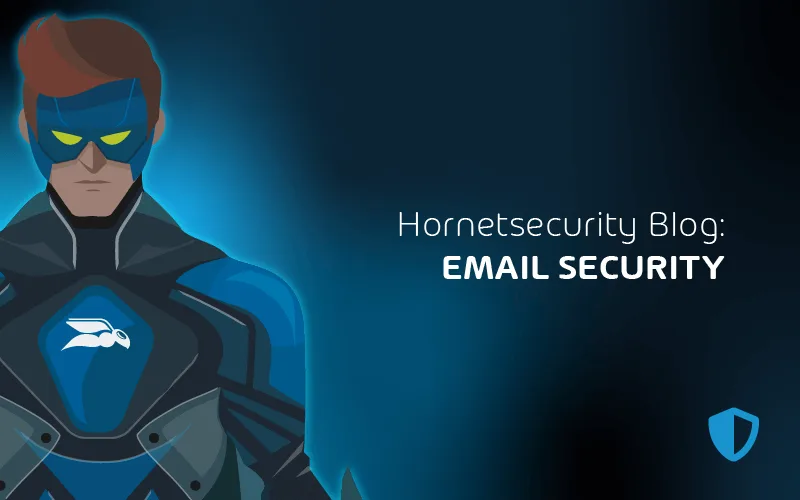
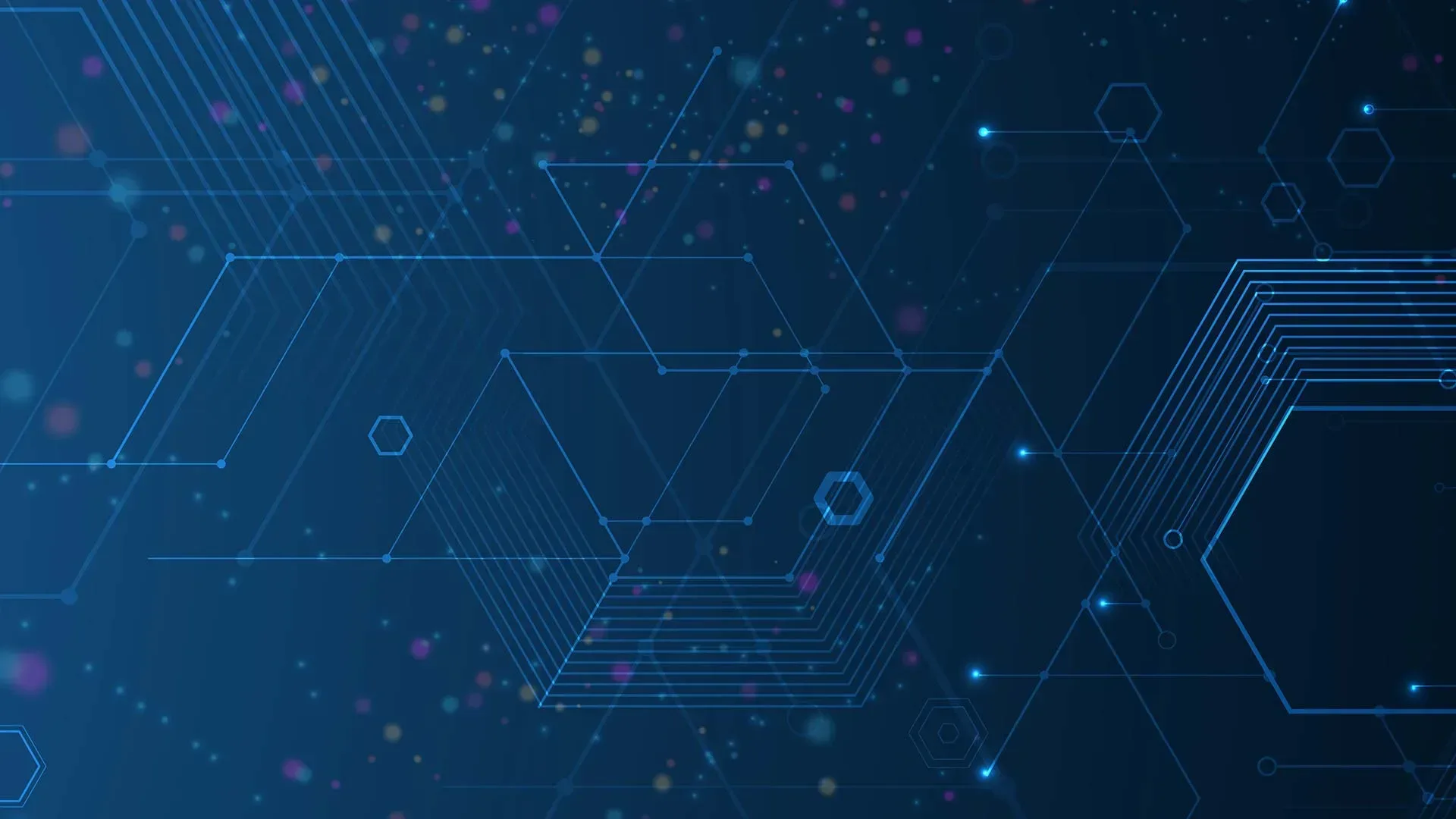
How AI is Revolutionizing Threat Detection
It’s no surprise to anyone reading this article that cyber security attacks are increasing in volume, sophistication and scope and at the same time, our businesses and societies are more dependent on digital infrastructure than ever.
But behind the scenes, often going completely unnoticed, is the application of AI threat detection technology, allowing us defenders to keep pace with the attackers. In this article, we’ll look at Machine Learning (ML) which is a subset of the larger Artificial Intelligence (AI) discipline and its applications in cybersecurity.
The importance of machine learning for threat detection
Most people know AI because of ChatGPT and its cousins but AI overall has been around since the 1960s and encompasses many areas such as Generative AI, Computer Vision, Autonomous Vehicles, Natural Language Processing, Voice Assistants, and more.
The main challenge for us defenders is the ease with which cyber-attacks can be mass-produced with slight variations, whether that’s a malicious program such as a virus or a worm, or different versions of a phishing email.
This makes it impossible to identify something “bad” because of what it is as it changes its appearance every time and instead forces us to spot it because of how it behaves, something that Machine Learning excels at.
Limitations of Traditional Threat Detection
Manual analysis is not sustainable
When I started in IT (a looong time ago) antivirus (AV) programs were driven by signatures.
Analysts would collect samples of real computer viruses, analyze them manually and produce a “fingerprint” so that the AV solution blocked them when they were downloaded or opened. As the number of new viruses per week grew from a handful to hundreds, then thousands this obviously wasn’t sustainable.
Don’t uninstall your AV however – just because there are newer threats, doesn’t mean old ones aren’t still used so a layered approach with different detection techniques working in unison is best.
New threats evolve faster than you can update
Another challenge with signature-based detections, or manually written rules is the detection lag. If you only update your signatures from the cloud every day, or a few times a day, there’s a time window where your system is vulnerable to recent threats.
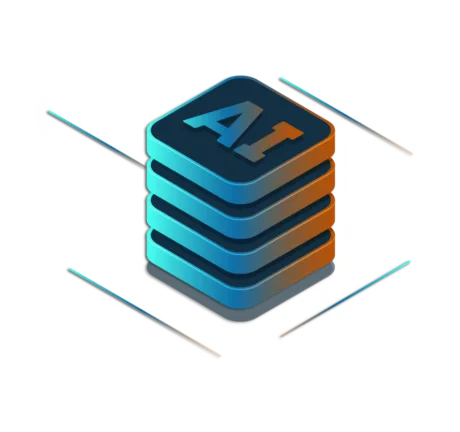
For endpoint protection specifically a turning point was the introduction of Endpoint Detection and Response (EDR), which tracks all process, file and registry activity on a system, providing rich log data for ML models to identify malicious activity.
How does AI enhance threat detection in cybersecurity?
To understand the power of ML for AI threat detection, let’s look at how it works. Take a huge amount of log data, the more the better, and mark malicious entries as such, and benign ones as good.
Feed that to the ML model which will then be exceptionally good at spotting new log data coming in that exhibit the characteristics it has learnt are associated with malicious activity. And unlike a human analyst, ML models will take into account many thousands different characteristics of each entry, weighting them and thus be able to spot anomalies that us humans wouldn’t be able to correlate.
The three main flavors of Machine Learning are:
- Supervised learning where you provide labeled training data as described above,
- Unsupervised learning where the model figures out the patterns by itself, and
- Reinforcement learning where you provide “rewards” when it gets it right, thus incentivizing it to find more ways of spotting the anomalies.
As new threat variants arise, we can tweak the models to be more efficient in their detections.
Sometimes these ML models run directly on the endpoint or system where the new log data is generated, other times they’re housed in the cloud where they have more underlying compute capacity and can be updated quicker.
And sometimes, both approaches are used – hey “this file you just downloaded and opened is acting a bit suspiciously, but I’m not sure, let me upload to the cloud for a final verdict from my more powerful ML brethren”.
And this brings us a system that’s not only looking at what something is to make a judgement about whether it’s bad or not, but also on how it behaves.
Challenges with AI threat detection
Because no ML system is perfect, the challenges with AI threat detection are false positives (FPs), where something is identified as dangerous when in fact it’s not, resulting in the blocking of legitimate business processes, plus the time and effort of manual intervention to fix it by IT / security staff.
There are also false negatives (FNs) where something was malicious but wasn’t identified as such, which of course is also not good.
Another benefit of using ML models for AI threat detection is that they can sometimes catch zero days, or a new attack that exploits a vulnerability in a system or application that the attackers are aware of, but the defenders aren’t.
Real-World Examples of AI & Machine Learning in Threat Detection
AI-driven threat detection is everywhere in cyber security, baked into services and solutions, quietly assisting us defenders:
Sentiment analysis with ChatGPT & Co.
A recent application of Artificial Intelligence in cybersecurity is using Generative AI (like Copilot or ChatGPT) to analyze the language used in emails and other messages. Here it’s less about identifying a malicious link and more about understanding the sentiment and tone of the message.
After all, if the attacker is trying to trick the user into changing an account number for an invoice payment, they’ll likely exchange a few emails back and forth to build up rapport and trust first, something the system will only understand if it’s analyzing the intent of the text itself, not just malicious links.
Network traffic analysis
Another area is network traffic analysis where vast amounts of log data is processed in real time, looking for anomalies and outliers. Even with most web traffic being protected by Transport Layer Security (TLS over the HTTPS protocol) there’s still a lot of metadata that can be used to identify malicious activity.
Authentication & Identity Protection
We’ve already mentioned protecting endpoints, another area that benefits from automated threat detection is authentication and identity protection.
All the large cloud providers have identity platforms, if you’re using Microsoft 365, that’s Entra ID, which receives over 7000 password attacks per second, along with many other attack attempts and uses ML to block attackers whilst allowing legitimate users to connect.
Attack Surface Management
Another interesting area is Attack Surface Management where unpatched and misconfigured services provide gaps where criminals can attack your organization. Finding all of those cracks and prioritizing which ones to attend to first is a prime use case for AI-driven security solutions.
Microsoft’s Threat Intelligence Tracking
In a similar way to the transition from signature-based AI for Antivirus to modern ML-powered EDR solutions, Microsoft has built Threat Intelligence Tracking via Dynamic Networks (TITAN).
Instead of analysts identifying IP addresses, URLs etc. as malicious manually from the huge pool of 78 trillion signals they collect daily, they use ML models to identify the relationship between entities and spot attacker infrastructure, which will be blocked in their security services, often before the attackers have had time to use it.
Threat Detection at Hornetsecurity
At Hornetsecurity we’ve been heavy users of AI threat detection and ML for many years, our Advanced Threat Protection service analyzes over 500 different characteristics of every email and attachments before it’s allowed into your end users inboxes, spotting badness with a very high degree of confidence.
We also use AI in our AI Recipient Validation service which ensures that your users don’t send emails to the wrong recipients by mistake.
More examples of generative AI in cybersecurity
Generative AI itself is also making its way into security products, Microsoft for example offers Copilot for Security as an add-on in their security products, allowing analysts to interact with security incidents, threat intelligence, and policies using natural language.
Don’t be surprised if your security services start offering chat-based interfaces for analyzing both threats and incidents, as well as feedback on existing policies and configurations.
Stay Ahead of Cyber Threats with AI-Driven Threat Detection
Machine learning is changing the game in cybersecurity. Faster detection, fewer false positives, and proactive threat prevention make AI essential for modern security.
Hornetsecurity’s Advanced Threat Protection solution use machine learning to keep your business secure.
- Real-time threat detection and analysis;
- Fewer false positives, faster response;
- Protection against zero-day attacks and APTs.
Discover how machine learning can strengthen your cybersecurity—schedule a demo today!
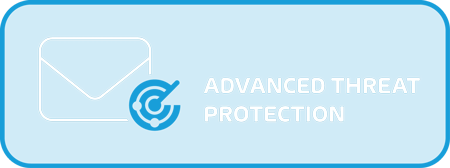
Conclusion – The Future of Threat Detection with Artificial Intelligence
There’s no doubt that AI and ML are here to stay in cyber security defense, there’s too much data, too many alerts and too many correlations for us humans to manage without them.
And apart from being able to handle gigantic amounts on log data, they’re also faster than us, and as cyber attackers are often moving from initial compromise of a business to complete takeover within hours instead of days or weeks, speed of response is increasingly important.
Hornetsecurity’s Advanced Threat Protection is a complete solution for email security, catching what others miss and keeping your business safe from the most important attack vector – email.
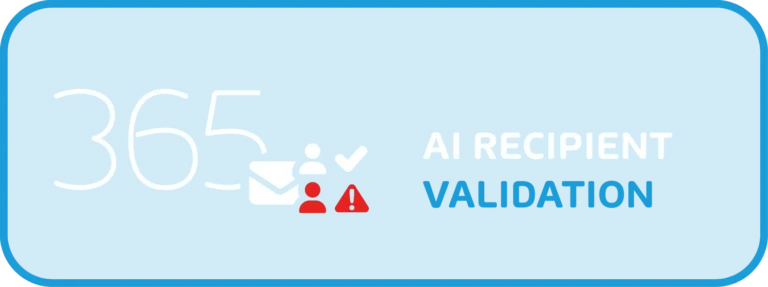
FAQ
AI enhances threat detection by utilizing Machine Learning (ML) to analyze vast amounts of log data. It identifies malicious behavior based on learned characteristics, enabling quicker and more accurate anomaly detection than human analysts.
The 3 main types of Machine Learning in threat detection include:
1. Supervised Learning, which uses labeled data;
2. Unsupervised Learning, which identifies patterns independently;
3. Reinforcement Learning, which incentivizes models to improve their detection capabilities.
ML models offer faster detection, reduced false positives, and the ability to identify zero-day attacks. They can analyze extensive data sets more efficiently than humans, significantly strengthening overall cybersecurity defenses.